
The
Data Story
In the following article, we tell the story of data using this infographic as a framework, and talk about how we work with clients to leverage their data assets for positive business change.
This article will grow over time as we publish each component of the story.
​
Keep an eye out for updates as we publish them here and on LinkedIn.
​
Follow us on LinkedIn to keep informed, or Contact Us to chat about your data challenges and opportunities.
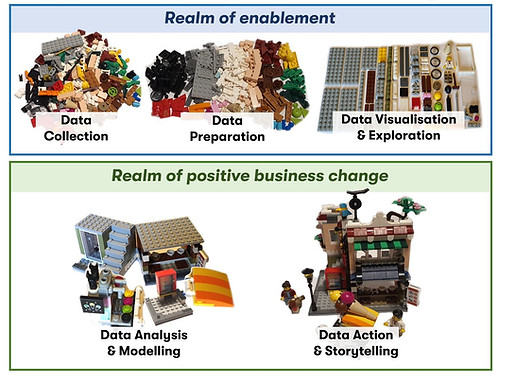
Part 2: Data Collection: Discovery
Part 3: Data Collection: Acquisition
Part 5: Data Visualisation and Exploration
​
Introduction
You may have seen something like this infographic before. We like it - not because it is searingly accurate, but because it is a simple aid to telling a story. As with any analogy, if you push it too hard it will fall over. However, with each section being meaningful, we can use it as a framework to tell the story of how we can help transform your business by turning your raw data into actionable, impactful insights to deliver positive business change.
Over the coming weeks, we will visit each stage in the journey from raw, unrefined data through to analysis, insight and action – using this simple device to tie the story together. We will talk about moving from the realm of enablement into the realm of positive business change and how you can make this happen. In this series of posts, we want to shed some light on how we help organisations at any level of data maturity make the best use of the data they have, and how this can be tied to business goals.
We will also be talking about the techniques, analyses, models and approaches we use to deliver real benefit in the realm of positive business change - the real game-changing things which sit within the Data Analysis & Modelling phase. We will talk about why a particular model or analysis can be of use, and how you can start to identify these opportunities within your business.
As we post updates to this series on LinkedIn, we will bring each element together here, so you can see the story building and how each of those elements fit together.
​
Data Collection
The Data Collection phase in the data story is concerned with two key concepts – Discovery and Acquisition. Discovery is the process through which we gain a deep understanding of our client’s business, the people involved with the processing and use of data, the current systems that perform that processing, the formal and informal processes in place and current and future requirements. Acquisition is the process of putting in place systems and process to gather each data set from around the business into a central location.
Using the analogy, you could imagine that this is process of asking your household where all the Lego sets are, going around and finding them, and then putting them all in a pile in the living room ready to sort.
Data Collection: Discovery
The importance of the discovery phase cannot be overstated – this part of the story lays the foundations on which the remaining parts are built. By diligently working with the client to really understand the what, where, who, why and when of data within the organisation, we can prepare all parties for the road ahead. In general there are five areas of work in the discovery phase that we concentrate on: People, Systems, Processes, Data and Access – however this may change depending on the size, type and maturity of the business. A key asset from the discovery process is a clear understanding of requirements – sometimes the client has a clear vision of what they want, and sometimes we partner with the business to help define that vision.
​
Data Collection: Acquisition
This phase is concerned with using all of the knowledge gained during the discovery phase to create a central “collection point” for all appropriate data. Early in the engagement the central collection point may be conceptual (a document listing all data sources, responsible people, processing rules, etc) and in later stages this may be materialised as an actual system (for example, a new cloud-based data storage facility with relevant ingress tooling). With modern tooling, this central collection point may remain “virtual” – i.e. data remains where it is, but becomes queryable from a single central location.
As part of the Acquisition process, we may be asked to recommend (and potentially install) a system that is appropriate for the task. In these instances we take a wide view of the market and recommend appropriate systems that fit the requirements and budget in place. We can also run RFI/RFP processes for our clients, asking the difficult questions to discover what is behind the shiny PowerPoint sales decks.
​
Data Collection: Discovery
In some cases, a first engagement with a new client may be a tightly bounded brief with specific deliverables and timelines. However if this is not the case, we always recommend starting new relationships with a discovery phase. This can lay the foundations for all future projects that leverage organisational data for positive business change. Although not imperative, it is incredibly useful to have an internal sponsor to work with who can make introductions to relevant people and help coordinate requests.
The objective of the discovery phase is to understand the key motivations behind the engagement, where the organisation is aiming to get to, and the key people, processes and systems that inform how data is currently used within the business.
For some organisations – particularly small businesses or those with high data maturity – the discovery phase can be fairly quick, as the scope may be narrow or documentation good. However, for other organisations this phase may take some time and will bring to the surface new insights into their people, systems, processes and data (valuable assets in their own right). Of course, one of the key insights from this work is discovering where the data actually is.
While we always seek to tailor our approach to discovery to the bespoke needs of the organisation we are working with, there are some key commonalities that feature in most discovery exercises.
​
Discovering People
Conducting a series of interviews with stakeholders and domain experts within the business will shed light on who owns what, who needs what, what challenges exist and the aspirations of teams and individuals when it comes to data and insight. It will also bring data champions to the fore – those who are already personally advancing up the data maturity ladder and who wish to bring their colleagues with them. These individuals are invaluable, as they can be key agents of change. From these interviews (and there may be many of them) a core group of people with the right knowledge and skills will emerge. This internal group will be critical in helping with the remaining aspects of discovery – and likely instrumental in delivery beyond the Discovery phase.
What we learn from these interviews will inform the remaining discovery exercise – systems, processes, data and access.
Discovering Systems
During the interviews, we aim to uncover as many of the business systems and linkages between them as possible. These will be mapped out and presented back, with data flows, ownership and responsibilities, external partners and suppliers, and other information attached. There can then be an iterative approach to completing the systems maps – once the internal teams see this new asset, it is likely to spark more conversations about which systems are in place and how they are connected and used (and what the opportunities and challenges are with them). Quite often there will be important components that are understood by a single person – and this may be the first time that many in the organisation have seen the full depth and breadth of systems in operation.
​
Discovering Processes
All organisations will have some processes in place to govern the implementation, development and use of their systems and data – whether these are well documented or live inside a single team member’s head. It is important that we pick out and document the processes that are pertinent to the data project – care should be taken here not to turn this effort into a full business process mapping exercise if that is not needed (for large organisations this can be a multi-month or multi-year project in its own right). We are mostly concerned with the processes that define how data systems operate, and how data flows into, through and out of the organisation. Where no process exists it may be necessary to help the organisation create one, particularly if it helps with good data governance.
The discovery of processes helps us to understand many aspects of the organisation’s operations, but it also helps to define how data is used and how it can be analysed and presented back to drive growth.
​
Discovering Data
Often the most complex part of discovery, data can be anywhere within an organisation. More often than not there will be a mix of well-governed and wild-west data – nicely configured and maintained databases alongside hundreds or thousands of individual, unmanaged and uncontrolled spreadsheets, CSV files and other structured and unstructured documents. During this part of discovery it is critical we focus on those data repositories and sources that are going to add value to the process. Often, a spreadsheet that a team member uses for their day-to-day work and would appear to be critical to them is derived from deeper, more “truthful” data sources – we need to find that source of the truth and work with it – how that team member then uses and manipulates that data for their own requirements needs to be understood, but this becomes more of a “process” than a data source.
​
Discovering Access
A common trip hazard in data projects is a lack of access to the systems and data required for discovery. During the interviews and development of the systems and process maps, we need to make sure that we find out how to gain access to those systems and data assets. This could be as simple as the provision of an endpoint, username and password or it may require more a more in-depth process where no direct access is provided and all requests for data are channelled through an internal team. Either way we work diligently with the security requirements of the organisation – we are being entrusted with access to the organisation’s crown jewels and always ensure we treat them as such. A security breach can spell serious trouble for both the organisation and us, so good governance is paramount.
​
Data Collection: Acquisition
Once we have discovered where the data is, what systems there are, who the relevant people in the organisation are and what processes and access requirements are in place, we can move on to the act of actually acquiring the data.
Acquisition is the final part of the “Collection” phase of the Data Story – and it may be surprising to learn that “acquisition” in this case doesn’t necessarily mean “gathering together”, or in fact moving the data at all. What we mean by acquisition is the process of making the data ready to “prepare” for analysis.
The process we go through for this depends partly on the data maturity of the organisation we are working with. Those that have a high level of data maturity may have great data environments, ready to use to process the data and prepare it for analysis – sometimes in-situ. For organisations that have a low level of data maturity, however, we may need to bring all of the data we have discovered into a new data environment to enable the preparation process to start. Sometimes this will necessitate the implementation of a temporary or permanent (depending on the type of engagement we have) system that will act as a central data store. Acquisition is then about bringing all of the relevant data into this new environment.
Either way, the outcome of the data acquisition process should be an environment that contains all relevant data (still quite raw), structured in a way that allows it to be prepared for analysis. This data preparation is then the next phase of the Data Story, which we’ll talk about next time.
​
Acquisition Methods
With thousands of different potential data sources, it is critical that we have experience with a good range of them. Acquiring data from a nicely constructed database might be relatively easy, however extracting tabular data from poorly scanned documents in PDF format presents some unique challenges.
Some common data acquisition methods are:
Querying structured files/databases
Making up the vast majority of data acquisition tasks, querying structured databases or files involves automated methods to extract data from systems using the relevant interfaces for those systems. Extracting large data sets from an existing database using SQL via a script is an example, as well as automatically processing 10,000 csv files stored in a document store and recognising and extracting appropriate data. Having a wide range of experience across many different types of datastore is critical – as well as the experience and skills to know how to extract that data in the most efficient way.
Web Scraping
Sometimes we find that an organisation has a critical but poorly maintained and documented application that they use to enter and store data. If there are no data export capabilities, then web scraping may be the only way to extract the data. If the application is not web based, then there are tools we can use to interact automatically with it in a scripted way and creatively extract the required data.
API interfaces
Where an API interface to an application is available, we can leverage that to extract data in a formal way. Sometimes this might require a bit of creativity to work with the limitations of the API, but usually there is a way! This requires an understating of the technology used for the API and how to construct efficient queries against it. APIs are sometimes “rate limited” (only allow a certain number of queries per second) or “response size” limited (will only return a response up to a maximum size) so for large extracts we may again need to be creative in how we construct the scripts.
PDF processing
May PDF files contain both the “image” of the document and the underlying text (which can easily be extracted) – but this isn’t always the case. Even if the underlying text can be extracted it’s usually not presented in a nice table (even though that is what the document shows). And if the text layer isn’t available then we need to train OCR (Optical Character Recognition) software to do the heavy lifting for us.
Manual Data Entry
It might sound archaic, but sometimes manual data entry is the best that’s available. If you have a cabinet of handwritten documents that need to be processed, it is sometimes much more efficient (and accurate) to transcribe them manually, compared with trying to configure a cutting edge scanning and text recognition system to do the job. Humans are still (currently) superior when context is key and content representing similar things is presented in different formats. There are many companies around the world that specialise in providing this service.
And many others
There are thousands of different ways that data can be stored, from hand-written documents to super-fast, niche, in-memory databases and they all have their idiosyncrasies. It is crucial that you have a data partner with the experience and creativity to deal with them all.
​
At the end of the Acquisition stage, we should have a well-documented set of data sources ready for processing. We may have moved some of the raw data into more appropriate locations, but some may be left where it is (if that location remains the most appropriate for that data). This documentation is now a hugely valuable asset for the organisation, and should be widely distributed particularly in the technical teams. It may contain information about the organisation’s data that has never before been seen and will support projects well beyond the current scope of work.
​
Data Preparation
The Data Preparation phase of the Data Story is concerned with taking all of the raw data we have now collected and preparing it for analysis. This phase can be quite lengthy, particularly if the data is messy, incomplete, sizable or contains known errors. However, the end result is a dataset that is magnitudes more useful than the raw data alone.
It is important to note that we do not totally replace the raw data with new prepared data – the original data should always remain available, even if we rarely delve into it. This availability can really help in a forensic understanding of why certain results have been achieved, and to provide a trail of truth that can be interrogated.
Data preparation is very wide subject, and we’ll look at just a few techniques and methods here. Often the process we will go through will depend on the data itself, it’s source, how it has been collected and a multitude of other factors.
Data Cleaning
One of the first steps in preparing the data for analysis is clean it appropriately. This is often one of the hardest stages as it deals with “unknown unknowns”, meaning that whether data is “clean” or not can in some cases be a little subjective. It takes years of experience handling data to identify which items within a data set need cleaning and then to define what “clean” actually means.
Key Data Cleaning items include:
-
Handling missing data
-
Handling outliers
-
Handling duplicate data
-
Handling inconsistent data
-
Formatting data
​
It's important to note that data cleaning process is iterative and requires a lot of attention to detail, it may require multiple rounds of cleaning and validation to ensure that the data is fit for the intended analysis.
Data Transformation
Data transformation, also known as data pre-processing or data wrangling, is the process of converting the data into a format that is appropriate for the intended analysis. The goal of this stage is to ensure that the data is in a format that can be easily used and understood by analysts and analytical systems, and that it is structured in a way that supports the intended analysis.
Key Data Transformation items include:
-
Data merging
-
Data normalisation
-
Data aggregation
-
Data filtering
-
Data enrichment
​
The data transformation process is iterative, and it may require multiple rounds of work ensure that the data is ready for the intended analysis. Also, for both transformation and cleaning it's important to keep track of the changes made to the original data set and to document the process, so that the results can be easily replicated and the process can be audited.
Data reduction
Data reduction, subsetting or summarisation is the process of reducing the size of the data set by selecting a subset of relevant data or by aggregating data at a higher level. The goal of this stage is to make the data set more manageable and easier to analyze, without compromising the integrity of the data. We generally have a very light touch in this area as we want to use as much of the data as possible for analysis and have designed our systems to process vert large datasets as required – however, there is always value in making sure the data is appropriately sized for the analysis at hand.
Key Data Reduction items include:
-
Sampling
-
Dimensionality reduction
-
Aggregation
-
Filtering
All of these approached slightly reduce the amount of information held within the data, so need to be applied with substantial care.
Data Validation
A crucial step in the preparation of data for analysis is to validate it. Data validation is the process of checking the data for errors, inconsistencies, or missing values, and ensuring that the data is complete and accurate. Optimally there will be a second source of data against which to validate the data – sometimes this can be something as basic as handwritten records, or someone’s understanding of the business. Often though, the validation requires the input of subject matter experts within a business to ascertain how “sensible” the data is. The goal of data validation is to ensure that the data is fit for purpose and can be used to make informed decisions.
Key Data Validation items include:
-
Quality checks (errors, inconsistencies etc)
-
Integrity checks
-
Completeness checks
-
Cross-checking
It's important to note that the data validation process should be done carefully, as it may affect the validity of the analysis results. Also, it's important to audit and keep track of the changes made to the original data set and to document the validation process, so that the results can be easily replicated and the process can be audited. Additionally, it's important to balance the need for data validation with the need for data completeness, so that the results of the analysis are accurate and reliable.
Data Preparation is the final stage before we start to explore and visualise the data, and that will be the point at which the client will start to see some novel insights as well as reflect some of what they already know. We know if the phases up to this point have been successful when the client starts to recognise some of the results of the initial visualisations – as they have a much deeper understanding of their business.
​
Data Visualisation and Exploration
The Data Visualisation and Exploration phase begins after the data has been cleaned and prepared. This is where we begin to make sense of the data and extract some preliminary insights.
It is important to note that visualisation and exploration are closely related, but distinct. Data visualisation is the process of creating graphical representations of data to make it more easily understandable and interpretable. By creating charts, graphs, maps, and other interactive forms of information delivery, we can assist our clients in identifying patterns, trends, and insights that would otherwise be difficult to discern from raw data. We utilise a variety of tools and techniques to create these visualisations, ensuring that they are easy to understand and interpret.
In contrast, data exploration is the act of actively engaging with the data to uncover new knowledge and trends. It involves a cyclical process of experimenting with various visualisations and examining different subsets of data. Techniques such as clustering, aggregation, and filtering are utilised to delve deeper into the data and uncover information that will aid in our subsequent analysis later down the line.
We use best practises for data visualisation and information delivery to help our clients get the most out of this stage. With our help in visualising and exploring data, organisations can identify patterns and trends that would otherwise be unnoticeable. That being said, the information obtained in this phase is primarily to fuel deeper analysis further down the line rather than to drive immediate business change – it is important to be aware of the significant hazards that come with using basic visualisations to inform substantial decisions.
Listed below are a variety of types of visualisations that allow clients to very quickly understand their data, and some of their appropriate use cases:
​
Line Charts
We utilise line charts to help our clients understand the trends and patterns in their time-series business data over time. Line charts are commonly used to display data such as sales, revenue, and customer engagement, making it easy for our clients to identify any fluctuations or trends in their data.
Bar Charts
Bar charts are an essential tool for our clients to compare values across different categories of business data. We use bar charts to display data such as market share, employee performance, and customer demographics, making it easy for our clients to conduct side-by-side comparisons of their categorical business data.
Heatmaps
Heatmaps are a powerful tool that we use to visualise our clients' business data in two dimensions and identify patterns and trends in their spatial data. We use heatmaps to display data such as customer location, store performance, and supply chain logistics, assisting our clients to uncover and discern patterns and correlations in their data through multiple variable analysis.
Scatter Plots
Scatter plots are also an excellent tool that we use to help our clients understand the relationships between different variables in their business data. We use scatter plots to display data such as customer demographics and purchase history, making it easy for our clients to identify patterns and trends in their data with multiple variables.
Pie Charts
We often utilise pie charts as a method for helping our clients comprehend the proportionality of various categorisations in their business data. We use these charts to showcase information such as sales of products, customer segmentation, and marketing campaign results, providing a simple way to view data from a relative perspective and directly compare different sections of data. Pie charts however are frequently misused, being less effective for comparing multiple values, but working well for illustrating one or two values in relation to the whole.
Some examples of basic exploration methods include, but are not restricted to:
Clustering
The process of grouping similar data points together based on their characteristics. This allows for the identification of patterns within the data, and can be used to group similar customers, products, or any other data points together. For example, clustering can be used to segment a customer base by demographics, purchase history, or any other relevant characteristics.
Aggregation
The process of combining data points to provide a higher-level summary of the data. This is typically done by taking the average, sum, or other aggregate function of a set of data points. For example, aggregation can be used to find the total sales of a particular product or the average customer satisfaction score across a certain time period, and for a range or dimensions.
Filtering
The process of selecting a subset of data based on certain criteria. This can be used to focus on specific aspects of the data that are of interest, such as a particular product or a specific time period.
Data visualisation and exploration are not just about creating appealing images. They are about turning data into insights that can help organisations achieve their goals. We work closely with our clients to understand their objectives and use visualisation and exploration techniques to help them reach them.